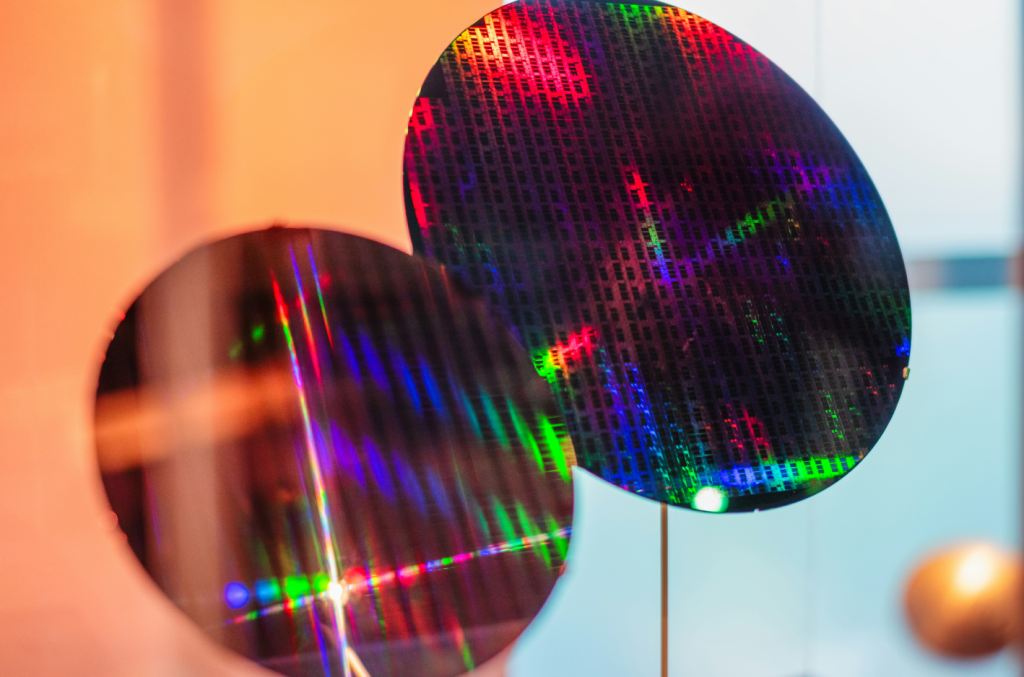
How to Start Delivering Value to End Customers Using AI
By: Mike Giles
24 October 2024
Introduction
This whitepaper is designed for C-suite executives and senior business leaders in financial institutions, providing a strategic framework for leveraging AI in customer-facing and front-office applications. As the financial services industry continues to evolve, AI presents an unprecedented opportunity to enhance customer engagement, streamline operations, and drive business value. However, the adoption of AI, particularly in customer-facing solutions, presents a unique set of challenges that require careful navigation.
As of Q4 2024, this whitepaper reflects insights developed by a team of enterprise technologists with deep experience in delivering bank-grade financial solutions and extensive knowledge of the regulatory and technical requirements unique to large financial institutions. We aim to fast-track your understanding of the current landscape of AI and offer practical advice on how to begin implementing AI in ways that protect your institution’s assets, maintain regulatory compliance, and deliver value to your customers.
AI Opportunities and Challenges for Financial Institutions
AI is a broad and transformative set of technologies that has made significant advancements in recent years. These technologies, when used correctly, can deliver substantial benefits for financial institutions, from improving operational efficiency to enhancing customer interactions. However, AI adoption has been uneven across the industry, with large enterprises—particularly financial institutions—lagging behind smaller, more agile players in the implementation of customer-facing AI solutions.
To date, AI has demonstrated its ability to drive efficiency in back-office operations, with applications such as predictive analytics, fraud detection, and process automation delivering measurable results. In contrast, the integration of AI into customer-facing applications has been slower, largely due to concerns around data privacy, intellectual property, and regulatory compliance.
Financial institutions face several key challenges when implementing AI in customer-facing solutions:
- Protection of Intellectual Property (IP): Ensuring that proprietary product information, algorithms, and processes remain secure and don’t ‘leak’ into third party AI engine providers and used to expand their knowledge is a top concern for financial institutions looking to integrate AI into their customer offerings.
- Customer Data Privacy: Financial institutions handle sensitive customer information, and AI implementations must ensure that this data is protected in compliance with strict regulatory requirements such as GDPR, HIPAA, and others. Even innocent customer conversations we don’t want accessed by third party AI providers.
- Accuracy, Reliability, and Fairness: AI models must be accurate, reliable, and free from bias. This requires transparent auditing mechanisms, clear accountability, and governance processes to ensure that AI-driven decisions can be trusted by both the institution and the customer.
- Governance and Oversight: Strong governance is essential to ensure that AI implementations adhere to the institution’s ethical, regulatory, and operational standards. Institutions must establish frameworks for AI oversight that include policies for auditing, transparency, and explainability.
Given these challenges, financial institutions must approach AI adoption with caution, focusing first on manageable, low-risk applications before scaling up to more complex, customer-facing use cases. In this whitepaper, we will discuss the current state of AI, explore the most promising use cases for customer-facing AI in financial services, and outline a step-by-step approach for integrating AI into your organisation.
Enterprise AI in 60 Seconds
At its core, AI can be broken down into several key categories, each with distinct applications and implications for financial institutions:
- Machine Learning (ML) and Big Data Analytics: These technologies allow financial institutions to derive insights from vast datasets, enabling more effective decision-making. Machine learning models are already widely used in back-office functions, including fraud detection, credit scoring, and predictive analytics. In these areas, AI has been instrumental in increasing efficiency and accuracy, providing institutions with valuable tools to enhance their operations.
- Speech Understanding and Synthesis: Advances in speech recognition and synthesis allow AI to power real-time, life-like voice interactions. These technologies are becoming increasingly sophisticated, enabling applications that can engage with customers through natural language processing (NLP). Think of this as a more advanced version of voice assistants like Siri or Alexa, but tailored to the specific needs of financial institutions.
- Generative AI: Generative AI represents a powerful new frontier for financial institutions, enabling the creation of new types of customer-facing applications. Generative AI leverages large language models (LLMs) to generate text, answer questions, and provide explanations based on vast amounts of data. These capabilities extend the functionality of business applications, allowing institutions to offer customers richer, more interactive experiences.
Generative AI models, such as OpenAI’s GPT, are trained on massive datasets that include everything from books and public websites to chat logs and private conversations. The accuracy and fairness of these models depend heavily on the quality of the underlying data. As a result, ensuring transparency around the provenance of data used to train these models is crucial for financial institutions implementing AI solutions. - Digital Content Generation (Images, Videos, Sound): AI has transformed the way digital content is created, allowing financial institutions to generate marketing materials, educational content, and personalised customer experiences with greater efficiency. Digital content generation tools can create everything from automated presentations to personalised investment reports, providing institutions with new ways to engage with their customers.
- Life-Like Avatars: AI-powered avatars are becoming increasingly realistic, offering financial institutions the ability to engage with customers in more human-like ways. These digital assistants can interact with customers through voice and video, offering personalised assistance and advice. Avatars can be embedded into websites and mobile applications, providing customers with a more engaging and interactive experience.
The technologies outlined above form the foundation of enterprise AI. While this is by no means an exhaustive list, it serves as a starting point for financial institutions looking to understand the AI landscape and begin their journey toward AI adoption.
An Incremental Approach to Deploying AI
Given the challenges of data privacy, intellectual property protection, and governance, most financial institutions have focused their AI efforts on back-office applications, where the risks are lower and the benefits more immediately apparent. Customer-facing AI use cases, on the other hand, have remained largely untapped, with only a few exceptions in areas such as personalised marketing and email content creation.
To successfully implement AI in customer-facing applications, financial institutions must take a measured, incremental approach. Rather than diving into the deep end, institutions should start with smaller, manageable projects that can be scaled up over time. This approach allows institutions to address key challenges—such as data privacy, IP protection, and governance—before deploying AI more broadly across the organisation.
Our recommendation for success is to start with manageable, controllable wins—use cases that deliver immediate value without exposing the institution to unnecessary risk. These use cases can be implemented incrementally, building on each other to create increasingly powerful, customer-facing AI solutions. By starting small and scaling up, financial institutions can ensure that their AI implementations are secure, compliant, and effective.
The Goal: Fully Personalised Digital Financial Assistants, Advisers, and Educators
Ultimately, the goal for financial institutions is to provide customers with fully personalised digital financial assistants. These assistants would be available 24/7, engaging with customers through natural language interactions and offering personalised advice, guidance, and support. They could be integrated across a range of financial applications, helping customers with everything from account management to investment planning.
In the long term, these digital financial assistants would have the following capabilities:
- Life-Like Avatars: The assistant would engage with customers through voice and video, providing a human-like experience.
- Rich, Contextual Conversations: The assistant would be able to offer personalised advice and guidance based on a deep understanding of the customer’s financial situation and goals.
To clarify how institutions can begin working towards this goal, we recommend breaking digital assistants into two levels of capability:
- Informational Assistants: These assistants would help customers understand information, guidance, and advice. This is where the industry is strongest today, and it represents a valuable starting point for institutions looking to implement AI in customer-facing applications.
- Actionable Assistants: These assistants would have access to transactional services, allowing them to look up personal customer data and execute transactions on behalf of the customer. While the technology to enable this level of capability exists, it requires robust governance and oversight to ensure that the AI operates within the institution’s ethical and regulatory frameworks.
By focusing on informational assistants first, financial institutions can deliver immediate value to customers while laying the groundwork for more advanced, actionable assistants in the future.
What AI is Capable of Today (Q4 2024)
As of Q4 2024, AI has demonstrated impressive capabilities in several key areas:
- Understanding Questions and Responding with Answers: AI’s natural language processing (NLP) capabilities have advanced significantly in recent years, enabling deep, meaningful conversations with users. AI can understand and respond to questions across multiple languages, as well as specialised languages such as programming code.
- Summarising Information: AI can summarise large volumes of information, providing users with concise, relevant answers based on both general and specific knowledge. This is particularly useful in financial applications, where customers often need quick access to complex information.
- Natural Voice and Speech: AI-generated voice has become much more natural and expressive, enabling more human-like interactions. Advances in text-to-speech technology have improved the quality of AI-generated speech, making it suitable for customer-facing applications.
- Digital Humans (Avatars): Digital avatars have become increasingly realistic, with the ability to sync voice and facial expressions to create a more engaging experience for users. While real-time conversation delivery still presents challenges, these avatars are becoming a valuable tool for financial institutions looking to enhance customer interactions.
- Multimodal AI: Emerging multimodal AI technologies allow users to interact with AI through a combination of text, sound, and video. This opens up new possibilities for customer engagement, allowing customers to interact with digital assistants that can “see” what they point their phone at, recognize objects, and respond accordingly.
- AI Agents: AI agents represent one of the most promising areas of AI development. These agents can be equipped with “tools” to access external data, execute transactions, and perform specific tasks. While AI agents have enormous potential for financial services, they also present significant governance and security challenges that institutions must address before deploying them in customer-facing applications.
Where to Start: Low-Hanging Use Cases with Immediate Value
For financial institutions looking to implement AI in customer-facing applications, the best place to start is by focusing on informational use cases. There is enormous value in better informing and educating customers about the institution’s products and services. A major barrier to financial product adoption is the customer’s lack of understanding, which often leads to incomplete transactions.
Research has shown that when customers receive human assistance, their likelihood of completing a transaction increases significantly. By integrating human-like digital assistants into financial applications, institutions can improve transaction completion rates and deliver a better customer experience.
Here are a few key areas where AI can be leveraged to deliver immediate value:
- AI Document Summarization: AI can summarise complex financial documents, providing customers with clear, concise explanations of key information.
- AI-Powered Avatars: Digital avatars can present these summaries and explanations in video format, offering a more engaging way for customers to interact with financial content.
- AI Chatbots: Smart chatbots can extend document summarization by answering customer questions and providing additional information in real time about the document and background concepts in the document.
By starting with these informational use cases, financial institutions can begin their AI journey with manageable, low-risk projects that deliver immediate value to customers.
Technical Building Blocks for AI Adoption
To successfully implement these AI use cases, financial institutions must focus on a few key technical building blocks:
- Enterprise AI Engine License: Institutions should acquire an enterprise-grade AI engine from providers like OpenAI, Meta, or AWS. These platforms offer increased privacy controls that ensure proprietary data is protected and not used to train third-party models.
- Retrieval Augmented Generation (RAG): RAG technology enhances the accuracy of out-of-the box AI engines whose vast general knowledge has been built upon massive amounts of publicly accessible text information, giving them great language skills but limits them to generic and sometimes out of date information sometime leading to the AI guessing and creating inaccurate answers called ‘hallucinations’. RAG techniques fill in these gaps by pulling real-time, organisation-specific knowledge from constantly updated specially structured knowledge bases. This approach gives the AI more specific context leading to more relevant responses to customer queries. RAG is an important technology for financial institutions as we can’t afford inaccuracies in the answers coming from our AI capabilities.
- Chatbot Capabilities: Based around a strong organisational knowledge base and RAG integration, financial institutions can deploy chatbots capable of handling complex, context-rich conversations with customers.
- Document Summarization Services: AI-powered document summarization can provide tailored summaries of complex documents, ensuring that customers have access to clear, understandable information.
- Digital Human Presenter Services: AI-powered avatars can deliver personalised, video-based content across a range of customer touchpoints, providing a more engaging and interactive experience for users.
- Voice Capabilities: Realistic voice chat is becoming possible and will be a key addition to the chatbot components once they become realistic enough and fast enough to maintain human level conversations. We are already close to this today.
- Audit Logging: – it is essential that we can record and log enough information about each step of any AI interaction including what the input request was, what the output was and also what the prompt and RAG context was sent to the model in each instance. This will allow us to verify answers and give explainability of the answers given by the AI. Scrubbing and redacting and PII for regional regulations.
- Accuracy testing: Random checking by humans and other intelligent processes can give comfort to our accuracy and consistency of answers.
By building out these technical foundations, financial institutions will be well-positioned to scale their AI capabilities over time, delivering increasingly sophisticated customer-facing solutions.
Governance and Oversight
As financial institutions integrate AI into their customer-facing applications, governance becomes a critical concern. Institutions must establish frameworks for AI oversight that ensure the accuracy, fairness, and transparency of AI-driven decisions.
Key governance considerations include:
- Data Provenance: Institutions must ensure that the data used to train AI models is legitimate and free from bias. This requires transparency around the sources of training data and ongoing monitoring to ensure compliance with ethical and regulatory standards.
- Audit and Compliance: AI systems must be subject to regular audits to ensure that they operate within the institution’s governance framework. This includes tracking decisions made by AI systems, monitoring for biases, and ensuring that AI-driven actions can be explained and justified.
- Privacy and Security: AI implementations must comply with data privacy regulations such as GDPR and HIPAA. This requires strict controls over how customer data is accessed, processed, and stored, as well as mechanisms to protect against unauthorised data usage.
By addressing these governance concerns, financial institutions can ensure that their AI implementations are secure, compliant, and trustworthy.
Conclusion
AI presents an unprecedented opportunity for financial institutions to enhance customer engagement and drive business value. By taking a cautious, incremental approach to AI adoption, institutions can deliver immediate value to their customers while laying the groundwork for more advanced AI capabilities in the future.
This whitepaper has outlined a framework for implementing AI in customer-facing applications, focusing on manageable, low-risk use cases that can be scaled over time. By starting with informational assistants and scaling up to more complex solutions, financial institutions can ensure that their AI implementations are secure, compliant, and effective.